About ACM Doctoral Dissertation Award
Presented annually to the author(s) of the best doctoral dissertation(s) in computer science and engineering. The Doctoral Dissertation Award is accompanied by a prize of $20,000, and the Honorable Mention Award is accompanied by a prize totaling $10,000. Winning dissertations will be published in the ACM Digital Library as part of the ACM Books Series.
Recent Doctoral Dissertation Award News
2024 ACM Doctoral Dissertation Award
ACM today announced that Ashish Sharma is the recipient of the ACM Doctoral Dissertation Award for his dissertation “Human-AI Collaboration to Support Mental Health and Well Being,” toward a PhD earned at the University of Washington. Sharma is a Senior Applied Scientist at the Microsoft Office of Applied Research.
Sharma developed fundamental advances in natural language processing to positively impact the mental health of many people. His approach involves constructing new machine learning models and algorithms that demonstrate psychological and societal understanding.
Importantly, Sharma’s work addresses the longstanding challenge that access to mental health services can be expensive. His most recent AI-supported mental health tool, for example, has been publicly deployed and used by a group of more than 160,000 people, the majority of whom are low-income. More than fifty percent of the people using these tools report a household income of less than $40,000 per year.
In developing these machine learning models and algorithms, Sharma incorporates principle-based ethical frameworks. Several mental health and technology organizations have adopted these tools, including Mental Health America and TalkLife.
Honorable Mentions for the ACM Doctoral Dissertation Award go to Alexander (Zander) Kelley for his dissertation “Explicit Pseudorandom Distributions for Restricted Models of Computation” toward a PhD earned at the University of Illinois Urbana-Champaign; and Sewon Min for her dissertation “Rethinking Data Use in Large Language Models” toward a PhD earned at the University of Washington.
2023 ACM Doctoral Dissertation Award
Nivedita Arora of Northwestern University is the recipient of the ACM Doctoral Dissertation Award for her dissertation “Sustainable Interactive Wireless Stickers: From Materials to Devices to Applications,” which demonstrated wireless and batteryless sensor nodes using novel materials and radio backscatter.
Arora’s research envisions creating sustainable computational materials that operate by harvesting energy from the environment and, at the end of their life cycle, can be responsibly composted or recycled. Her research process involves working at the intersection of materials, methods of fabrication, low-power systems, and HCI . She actively looks to apply her work to application domains such as smart homes, health, climate change, and wildlife monitoring.
Arora’s dissertation makes truly groundbreaking contributions to the fields of Ubiquitous Computing and Human-Computer Interaction. Today’s Internet of Things (IoT) devices are bulky, require battery maintenance, and involve costly installation. In contrast, Arora shows how the computational capabilities of sensing, communication, and display can be diffused into materials and everyday objects. She builds interactive stickers that are inexpensive, and easy to deploy and sustainably operate by harvesting energy from body heat or indoor light. She demonstrates this idea over a series of projects. Her first effort, SATURN, is a thin, flexible multi-layer material that is a self-sustaining audio sensor. Specifically, it uses the vibration itself to power the ability to capture and encode the vibration sensor. SATURN was extended to ZEUSSS to use passive RF backscatter for wireless transmission on the vibration signal. She followed this up with the MARS platform that produces an extremely low-power (less than a microwatt) resonance circuit that varies its frequency based on user interaction with interfaces that create inductive or capacitive loads on the circuit. Coupling this circuit with FM passive backscatter and ambient power harvesting allows user interfaces such as touch-sensitive buttons, sliders, and vibration sensors to communicate at a distance. The result of these three projects is a flat user interface in a post-it note form factor that can be deployed in the environment simply by sticking it to a flat surface. The flat user interface and mobile design allows for applications such as light switches or audio volume sliders that can simply be pasted where they are needed without worrying about wiring the infrastructure or maintaining batteries.
The final project, VENUS, adds output in the form of low-power display technologies to provide immediate feedback on the surface of the computational material, opening a wide variety of user-facing interaction scenarios. Her work also showed that it is possible to power these circuits through the transfer of body heat when a user touches the button, which can also be used to protect privacy.
Arora is an Assistant Professor in the Electrical and Computer Engineering and (by courtesy) Computer Science Department, as well as the Allen K. and Johnnie Cordell Breed Jr. Professor of Design at Northwestern University. Her research involves rethinking the computing stack from a sustainability-first approach for its entire life-cycle: manufacturing, operation, and disposal. Arora received a PhD in Computer Science and an MS In Human-Computer Interaction from the Georgia Institute of Technology.
Honorable Mentions
Honorable Mentions for the ACM Doctoral Dissertation Award go to Gabriele Farina of the Massachusetts Institute of Technology, and William Kuszmaul of Harvard University.
Farina’s dissertation, “Game-Theoretic Decision Making in Imperfect-Information Games” was recognized for laying modern learning foundations for decision-making in imperfect-information sequential games, resolving long-standing questions, and demonstrating state-of-the-art theoretical and practical performance.
Farina is an Assistant Professor in the Electrical Engineering and Computer Science Department (EECS) at the Massachusetts Institute of Technology. His research interests include artificial intelligence, machine learning, optimization, and game theory. He received a PhD in Computer Science from Carnegie Mellon University.
Kuszmaul’s dissertation, “Randomized Data Structures: New Perspectives and Hidden Surprises,” is recognized for contributions to the field of randomized data structures that overturn conventional wisdom and widely believed conjecture.
Kuszmaul’s research focuses on algorithms, data structures, and probability. He received a PhD in Computer Science from the Massachusetts Institute of Technology and is presently doing Post Doctoral work at Harvard University. In August, he will be starting as an assistant professor in the Computer Science Department at Carnegie Mellon University.
2022 ACM Doctoral Dissertation Award
Aayush Jain is the recipient of the 2022 ACM Doctoral Dissertation Award for his dissertation “Indistinguishability Obfuscation From Well-Studied Assumptions,” which established the feasibility of mathematically rigorous software obfuscation from well-studied hardness conjectures.
The central goal of software obfuscation is to transform source code to make it unintelligible without altering what it computes. Additional conditions may be added, such as requiring the transformed code to perform similarly, or even indistinguishably, from the original. As a software security mechanism, it is essential that software obfuscation have a firm mathematical foundation.
The mathematical object that Jain’s thesis constructs, indistinguishability obfuscation, is considered a theoretical “master tool” in the context of cryptography—not only in helping achieve long-desired cryptographic goals such as functional encryption, but also in expanding the scope of the field of cryptography itself. For example, indistinguishability obfuscation aids in goals related to software security that were previously entirely in the domain of software engineering.
Jain’s dissertation was awarded the Best Paper Award at the ACM Symposium on Theory of Computing (STOC 2021) and was the subject of an article in Quanta Magazine titled “Scientists Achieve Crown Jewel of Cryptography.”
Jain is an Assistant Professor at Carnegie Mellon University. He is interested in theoretical and applied cryptography and its connections with related areas of theoretical computer science. Jain received a BTech in Electrical Engineering, and an MTech in Information and Communication Technology from the Indian Institute of Technology, Delhi. He received a PhD in Computer Science from the University of California, Los Angeles.
Honorable Mentions
Honorable Mentions for the 2022 ACM Doctoral Dissertation Award go to Alane Suhr whose PhD was earned at Cornell University, and Conrad Watt, who earned his PhD at the University of Cambridge.
Suhr’s dissertation, “Reasoning and Learning in Interactive Natural Language Systems,” was recognized for formulating and designing algorithms for continual language learning in collaborative interactions, and designing methods to reason about context-dependent language meaning. Suhr’s dissertation made transformative contributions in several areas of Natural Language Processing (NLP).
Suhr is an Assistant Professor at the University of California, Berkeley. Suhr’s research is focused on natural language processing, machine learning, and computer vision. Suhr received a BS in Computer Science and Engineering from Ohio State University, as well as a PhD in Computer Science from Cornell University.
Watt’s dissertation, “Mechanising and Evolving the Formal Semantics of WebAssembly: the Web’s New Low-Level Language,” establishes a mechanized semantics for WebAssembly and defines its concurrency model. The model will underpin current and future web engineering. His dissertation is considered a stand-out example of developing and using fully rigorous mechanized semantics to directly affect and improve the designs of major pieces of our industrial computational infrastructure.
Watt is a Research Fellow (postdoctoral) at the University of Cambridge, where he focuses on mechanized formal verification, concurrency, and the WebAssembly language. He received a MEng in Computer Science from Imperial College London and a PhD in Computer Science from the University of Cambridge.
2021 ACM Doctoral Dissertation Award
Manish Raghavan is the recipient of the 2021 ACM Doctoral Dissertation Award for his dissertation "The Societal Impacts of Algorithmic Decision-Making." Raghavan’s dissertation makes significant contributions to the understanding of algorithmic decision making and its societal implications, including foundational results on issues of algorithmic bias and fairness.
Algorithmic fairness is an area within AI that has generated a great deal of public and media interest. Despite being at a very early stage of his career, Raghavan has been one of the leading figures shaping the direction and focus of this line of research.
Raghavan is a Postdoctoral Fellow at the Harvard Center for Research on Computation and Society. His primary interests lie in the application of computational techniques to domains of social concern, including algorithmic fairness and behavioral economics, with a particular focus on the use of algorithmic tools in the hiring pipeline. Raghavan received a BS degree in Electrical Engineering and Computer Science from the University of California, Berkeley, and MS and PhD degrees in Computer Science from Cornell University.
Honorable Mentions for the 2021 ACM Doctoral Dissertation Award go to Dimitris Tsipras of Stanford University, Pratul Srinivasan of Google Research and Benjamin Mildenhall of Google Research.
Dimitris Tsipras’ dissertation, “Learning Through the Lens of Robustness,” was recognized for foundational contributions to the study of adversarially robust machine learning (ML) and building effective tools for training reliable machine learning models. Tsipras made several pathbreaking contributions to one of the biggest challenges in ML today: making ML truly ready for real-world deployment.
Tsipras is a Postdoctoral Scholar at Stanford University. His research is focused on understanding and improving the reliability of machine learning systems when faced with the real world. Tsipras received a Diploma in Electrical and Computer Engineering from the National Technical University of Athens, as well as SM and PhD degrees in computer science from the Massachusetts Institute of Technology (MIT).
Pratul Srinivasan and Benjamin Mildenhall are awarded Honorable Mentions for their co-invention of the Neural Radiance Field (NeRF) representation, associated algorithms and theory, and their successful application to the view synthesis problem. Srinivasan’s dissertation, "Scene Representations for View Synthesis with Deep Learning," and Mildenhall’s dissertation, “Neural Scene Representations for View Synthesis,” addressed a long-standing open problem in computer vision and computer graphics. That problem, called “view synthesis” in vision and “unstructured light field rendering” in graphics, involves taking just a handful of photographs of a scene and predicting new images from any intermediate viewpoint. NeRF has already inspired a remarkable volume of follow-on research, and the associated publications have received some of the fastest rates of citation in computer graphics literature—hundreds in the first year of post-publication.
Srinivasan is a Research Scientist at Google Research, where he focuses on problems at the intersection of computer vision, computer graphics, and machine learning. He received a BSE degree in Biomedical Engineering and BA in Computer Science from Duke University and a PhD in Computer Science from the University of California, Berkeley.
Mildenhall is a Research Scientist at Google Research, where he works on problems in computer vision and graphics. He received a BS degree in Computer Science and Mathematics from Stanford University and a PhD in Computer Science from the University of California, Berkeley.
2020 ACM Doctoral Dissertation Award
Chuchu Fan is the recipient of the 2020 ACM Doctoral Dissertation Award for her dissertation, “Formal Methods for Safe Autonomy: Data-Driven Verification, Synthesis, and Applications.” The dissertation makes foundational contributions to verification of embedded and cyber-physical systems, and demonstrates applicability of the developed verification technologies in industrial-scale systems.
Fan’s dissertation also advances the theory for sensitivity analysis and symbolic reachability; develops verification algorithms and software tools (DryVR, Realsyn); and demonstrates applications in industrial-scale autonomous systems.
Key contributions of her dissertation include the first data-driven algorithms for bounded verification of nonlinear hybrid systems using sensitivity analysis. A groundbreaking demonstration of this work on an industrial-scale problem showed that verification can scale. Her sensitivity analysis technique was patented, and a startup based at the University of Illinois at Urbana-Champaign has been formed to commercialize this approach.
Fan also developed the first verification algorithm for “black box” systems with incomplete models combining probably approximately correct (PAC) learning with simulation relations and fixed point analyses. DryVR, a tool that resulted from this work, has been applied to dozens of systems, including advanced driver assist systems, neural network-based controllers, distributed robotics, and medical devices.
Additionally, Fan’s algorithms for synthesizing controllers for nonlinear vehicle model systems have been demonstrated to be broadly applicable. The RealSyn approach presented in the dissertation outperforms existing tools and is paving the way for new real-time motion planning algorithms for autonomous vehicles.
Fan is the Wilson Assistant Professor of Aeronautics and Astronautics at the Massachusetts Institute of Technology, where she leads the Reliable Autonomous Systems Lab. Her group uses rigorous mathematics including formal methods, machine learning, and control theory for the design, analysis, and verification of safe autonomous systems. Fan received a BA in Automation from Tsinghua University. She earned her PhD in Electrical and Computer Engineering from the University of Illinois at Urbana-Champaign.
Honorable Mentions for the 2020 ACM Doctoral Dissertation Award go to Henry Corrigan-Gibbs and Ralf Jung.
Corrigan-Gibbs’s dissertation, “Protecting Privacy by Splitting Trust,” improved user privacy on the internet using techniques that combine theory and practice. Corrigan-Gibbs first develops a new type of probabilistically checkable proof (PCP), and then applies this technique to develop the Prio system, an elegant and scalable system that addresses a real industry need. Prio is being deployed at several large companies, including Mozilla, where it has been shipping in the nightly version of the Firefox browser since late 2019, the largest-ever deployment of PCPs.
Corrigan-Gibbs’s dissertation studies how to robustly compute aggregate statistics about a user population without learning anything else about the users. For example, his dissertation introduces a tool enabling Mozilla to measure how many Firefox users encountered a particular web tracker without learning which users encountered that tracker or why. The thesis develops a new system of probabilistically checkable proofs that lets every browser send a short zero-knowledge proof that its encrypted contribution to the aggregate statistics is well formed. The key innovation is that verifying the proof is extremely fast.
Corrigan-Gibbs is an Assistant Professor in the Electrical Engineering and Computer Science Department at the Massachusetts Institute of Technology, where he is also a member of the Computer Science and Artificial Intelligence Lab. His research focuses on computer security, cryptography, and computer systems. Corrigan-Gibbs received his PhD in Computer Science from Stanford University.
Ralf Jung’s dissertation, “Understanding and Evolving the Rust Programming Language,” established the first formal foundations for safe systems programming in the innovative programming language Rust. In development at Mozilla since 2010, and increasingly popular throughout the industry, Rust addresses a longstanding problem in language design: how to balance safety and control. Like C++, Rust gives programmers low-level control over system resources. Unlike C++, Rust also employs a strong “ownership-based” system to statically ensure safety, so that security vulnerabilities like memory access errors and data races cannot occur. Prior to Jung’s work, however, there had been no rigorous investigation of whether Rust’s safety claims actually hold, and due to the extensive use of “unsafe escape hatches” in Rust libraries, these claims were difficult to assess.
In his dissertation, Jung tackles this challenge by developing semantic foundations for Rust that account directly for the interplay between safe and unsafe code. Building upon these foundations, Jung provides a proof of safety for a significant subset of Rust. Moreover, the proof is formalized within the automated proof assistant Coq and therefore its correctness is guaranteed. In addition, Jung provides a platform for formally verifying powerful type-based optimizations, even in the presence of unsafe code.
Through Jung's leadership and active engagement with the Rust Unsafe Code Guidelines working group, his work has already had profound impact on the design of Rust and laid essential foundations for its future.
Jung is a post-doctoral researcher at the Max Planck Institute for Software Systems and a research affiliate of the Parallel and Distributed Operating Systems Group at the Massachusetts Institute of Technology. His research interests include programming languages, verification, semantics, and type systems. He conducted his doctoral research at the Max Planck Institute for Software Systems, and received his PhD, Master's, and Bachelor's degrees in Computer Science from Saarland University.
2019 ACM Doctoral Dissertation Award
Dor Minzer of Tel Aviv University is the recipient of the 2019 ACM Doctoral Dissertation Award for his dissertation, “On Monotonicity Testing and the 2-to-2-Games Conjecture.” The key contributions of Minzer’s dissertation are settling the complexity of testing monotonicity of Boolean functions and making a significant advance toward resolving the Unique Games Conjecture, one of the most central problems in approximation algorithms and complexity theory.
Property-testers are extremely efficient randomized algorithms that check whether an object satisfies a certain property, when the data is too large to examine. For example, one may want to check that the distance between any two computers in the internet network does not exceed a given bound. In the first part of his thesis, Minzer settled a famous open problem in the field by introducing an optimal tester that checks whether a given Boolean function (voting scheme) is monotonic.
The holy grail of complexity theory is to classify computational problems to those that are feasible and those that are infeasible. The PCP theorem (for probabilistically checkable proofs) establishes the framework that enables classifying approximation problems as infeasible, showing they are NP-hard. In 2002, Subhash Khot proposed the Unique Games Conjecture (UGC), asserting that a very strong version of the PCP theorem should still hold. The conjecture has inspired a flurry of research and has had far-reaching implications. If proven true, the conjecture would explain the complexity of a whole family of algorithmic problems. In contrast to other conjectures, UGC has been controversial, splitting the community into believers and skeptics. While progress toward validating the conjecture has stalled, evidence against it had been piling up, involving new algorithmic techniques.
In the second part of his dissertation, Minzer went halfway toward establishing the conjecture, and in the process nullified the strongest known evidence against UGC. Even if UGC is not resolved in the immediate future, Minzer’s dissertation makes significant advances toward solving research problems that have previously appeared out of reach.
Minzer is a postdoctoral researcher at the Institute for Advanced Study (IAS) in Princeton, New Jersey, and will be joining MIT as an Assistant Professor in the fall of 2020. His main research interests are in computational complexity theory, PCP, and analysis of Boolean functions. Minzer received a BA in Mathematics, as well as an MSc and PhD in Computer Science from Tel Aviv University.
Honorable Mentions for the 2019 ACM Doctoral Dissertation Award go to Jakub Tarnawski, École polytechnique fédérale de Lausanne (EPFL) and JiaJun Wu, Massachusetts Institute of Technology (MIT).
Jakub Tarnawski’s dissertation “New Graph Algorithms via Polyhedral Techniques” made groundbreaking algorithmic progress on two of the most central problems in combinatorial optimization: the matching problem and the traveling salesman problem. Work on deterministic parallel algorithms for the matching problem is motivated by one of the unsolved mysteries in computer science: does randomness help in speeding up algorithms? Tarnawski’s dissertation makes significant progress on this question by almost completely derandomizing a three-decade-old randomized parallel matching algorithm by Ketan Mulmuley, Umesh Vaziriani, and Vijay Vazirani.
The second major result of Tarnawski’s dissertation relates to the traveling salesman problem: find the shortest tour of n given cities. Already in 1956, George Dantzig et al. used a linear program to solve a special instance of the problem. Since then the strength of their linear program has become one of the main open problems in combinatorial optimization. Tarnawski’s dissertation resolves this question asymptotically and gives the first constant-factor approximation algorithm for the asymmetric traveling salesman problem.
Tarnawski is a researcher at Microsoft Research. He is broadly interested in theoretical computer science and combinatorial optimization, particularly in graph algorithms and approximation algorithms. He received his PhD from EPFL and an MSc in Mathematics and Computer Science from the University of Wrocław, Poland.
JiaJun Wu’s dissertation, “Learning to See the Physical World,” has advanced AI for perceiving the physical world by integrating bottom-up recognition in neural networks with top-down simulation engines, graphical models, and probabilistic programs. Despite phenomenal progress in the past decade, current artificial intelligence methods tackle only specific problems, require large amounts of training data, and easily break when generalizing to new tasks or environments. Human intelligence reveals how far we need to go: from a single image, humans can explain what we see, reconstruct the scene in 3D, predict what’s going to happen, and plan our actions accordingly.
Wu addresses the problem of physical scene understanding—how to build efficient and versatile machines that learn to see, reason about, and interact with the physical world. The key insight is to exploit the causal structure of the world, using simulation engines for computer graphics, physics, and language, and to integrate them with deep learning. His dissertation spans perception, physics and reasoning, with the goal of seeing and reasoning about the physical world as humans do. The work bridges the various disciplines of artificial intelligence, addressing key problems in perception, dynamics modeling, and cognitive reasoning.
Wu is an Assistant Professor of Computer Science at Stanford University. His research interests include physical scene understanding, dynamics models, and multi-modal perception. He received his PhD and SM degree in Electrical Engineering and Computer Science from MIT, and Bachelor’s degrees in Computer Science and Economics from Tsinghua University in Beijing, China.
2018 ACM Doctoral Dissertation Award
Chelsea Finn of the University of California, Berkeley is the recipient of the 2018 ACM Doctoral Dissertation Award for her dissertation, “Learning to Learn with Gradients.” In her thesis, Finn introduced algorithms for meta-learning that enable deep networks to solve new tasks from small datasets, and demonstrated how her algorithms can be applied in areas including computer vision, reinforcement learning and robotics.
Deep learning has transformed the artificial intelligence field and has led to significant advances in areas including speech recognition, computer vision and robotics. However, deep learning methods require large datasets, which aren’t readily available in areas such as medical imaging and robotics.
Meta-learning is a recent innovation that holds promise to allow machines to learn with smaller datasets. Meta-learning algorithms “learn to learn” by using past data to learn how to adapt quickly to new tasks. However, much of the initial work in meta-learning focused on designing increasingly complex neural network architectures. In her dissertation, Finn introduced a class of methods called model-agnostic meta-learning (MAML) methods, which don’t require computer scientists to manually design complex architectures. Finn’s MAML methods have had tremendous impact on the field and have been widely adopted in reinforcement learning, computer vision and other fields of machine learning.
At a young age, Finn has become one of the most recognized experts in the field of robotic learning. She has developed some of the most effective methods to teach robots skills to control and manipulate objects. In one instance highlighted in her dissertation, she used her MAML methods to teach a robot reaching and placing skills, using raw camera pixels from just a single human demonstration.
Finn is a Research Scientist at Google Brain and a postdoctoral researcher at the Berkeley AI Research Lab (BAIR). In the fall of 2019, she will start a full-time appointment as an Assistant Professor at Stanford University. Finn received her PhD in Electrical Engineering and Computer Science from the University of California, Berkeley and a BS in Electrical Engineering and Computer Science from the Massachusetts Institute of Technology.
Honorable Mentions for the 2018 ACM Doctoral Dissertation Award go to Ryan Beckett and Tengyu Ma, who both received PhD degrees in Computer Science from Princeton University.
Ryan Beckett developed new, general and efficient algorithms for creating and validating network control plane configurations in his dissertation, “Network Control Plane Synthesis and Verification.” Computer networks connect key components of the world’s critical infrastructure. When such networks are misconfigured, several systems people rely on are interrupted—airplanes are grounded, banks go offline, etc. Beckett’s dissertation describes new principles, algorithms and tools for substantially improving the reliability of modern networks. In the first half of his thesis, Beckett shows that it is unnecessary to simulate the distributed algorithms that traditional routers implement—a process that is simply too costly—and that instead, one can directly verify the stable states to which such algorithms will eventually converge. In the second half of his thesis, he shows how to generate correct configurations from surprisingly compact high-level specifications.
Beckett is a researcher in the mobility and networking group at Microsoft Research. He received his PhD and MA in Computer Science from Princeton University, and both a BS in Computer Science and a BA in Mathematics from the University of Virginia.
Tengyu Ma’s dissertation, "Non-convex Optimization for Machine Learning: Design, Analysis, and Understanding,” develops novel theory to support new trends in machine learning. He introduces significant advances in proving convergence of nonconvex optimization algorithms in machine learning, and outlines properties of machine learning models trained via such methods. In the first part of his thesis, Ma studies a range of problems, such as matrix completion, sparse coding, simplified neural networks, and learning linear dynamical systems, and formalizes clear and natural conditions under which one can design provable correct and efficient optimization algorithms. In the second part of his thesis, Ma shows how to understand and interpret the properties of embedding models for natural languages, which were learned using nonconvex optimization.
Ma is an Assistant Professor of Computer Science and Statistics at Stanford University. He received a PhD in Computer Science from Princeton University and a BS in Computer Science from Tsinghua University.
2017 ACM Doctoral Dissertation Award
Aviad Rubinstein is the recipient of the Association for Computing Machinery (ACM) 2017 Doctoral Dissertation Award for his dissertation “Hardness of Approximation Between P and NP.” In his thesis, Rubinstein established the intractability of the approximate Nash equilibrium problem and several other important problems between P and NP-completeness—an enduring problem in theoretical computer science.
For several decades, researchers in areas including economics and game theory have developed mathematical equilibria models to predict how people in a game or economic environment might act given certain conditions.
When applying computational approaches to equilibria models, important questions arise, including how long it would take a computer to calculate an equilibrium. In theoretical computer science, a problem that can be solved in theory (given finite resources, such as time) but for which, in practice, any solution takes too many resources (that is, too much time) to be useful is known as an intractable problem. In 2008, Daskalakis, Goldberg and Papadimitriou demonstrated the intractability of the Nash equilibrium, an often-examined scenario in game theory and economics where no player in the game would take a different action as long as every other player in the game remains the same. But a very large question remained in theoretical computer science as to whether an approximate Nash equilibrium (a variation of the Nash equilibrium that allows the possibility that a player may have a small incentive to do something different) is also intractable.
Rubinstein’s dissertation introduced brilliant new ideas and novel mathematical techniques to demonstrate that the approximate Nash equilibrium is also intractable. Beyond solving this important question, Rubinstein’s thesis also insightfully addressed other problems around P and NP completeness, the most important question in theoretical computer science. Rubinstein is a postdoctoral researcher at Harvard University and will be starting an appointment as an Assistant Professor at Stanford University in the fall of 2018. He received a PhD in Computer Science from the University of California, Berkeley, an MSc in Computer Science from Tel Aviv University (Israel) and a BSc in Mathematics and Computer Science from Technion (Israel).
Honorable Mentions for the 2017 ACM Doctoral Dissertation Award went to Mohsen Ghaffari, who received his PhD from the Massachusetts Institute of Technology’s Department of Electrical Engineering and Computer Science (MIT EECS) and Stefanie Mueller, who received her PhD from the Hasso Plattner Institute (Germany).
In Ghaffari’s dissertation, “Improved Distributed Algorithms for Fundamental Graph Problems,” he presents novel distributed algorithms that significantly lower the costs of solving fundamental graph problems in networks, including structuring problems, connectivity problems, and scheduling problems. Ghaffari’s dissertation includes both breakthrough algorithmic contributions and interesting methodology. The first part of the dissertation presents a new maximal independent set (MIS) algorithm, which is a breakthrough because it achieves a better time bound than previous algorithms for this three-decades-old problem. The second part of the dissertation contains a collection of related results about vertex connectivity decompositions. Finally, in the third part of his dissertation, Ghaffari introduces a time-efficient algorithm for concurrent scheduling of multiple distributed algorithms. Ghaffari is an Assistant Professor of Computer Science at ETH Zurich. He received a PhD and SM in Electrical Engineering and Computer Science from the Massachusetts Institute of Technology and received a double major in Computer Science and Electrical Engineering from Sharif University (Iran).
Mueller’s dissertation, “Interacting with Personal Fabrication Devices,” demonstrates how to make personal fabrication machines interactive. Her approach involves two steps: speeding of batch processing and turn taking, and real-time interaction. Her software systems faBrickator, WirePrint and Platener allow users to fabricate 10 times faster, a process she calls low-fidelity fabrication or low-fab. In her dissertation she also outlines how to add interactivity. Constructable, a tool she developed, allows workers to fabricate by sketching directly on the workpiece, causing a laser cutter to implement these sketches when the user stops drawing. Another of Mueller’s tools, LaserOrigami, extends this work to 3D. Mueller is an Assistant Professor of Computer Science at MIT EECS and MIT CSAIL. She received a PhD in Computer Science as well as an MSc in IT-Systems Engineering from the Hasso Plattner Institute (Germany). Earlier, she received a BSc in Computer Science and Media from the University of Applied Science Harz (Germany).
2016 ACM Doctoral Dissertation Award
Haitham Hassanieh is the recipient of the ACM 2016 Doctoral Dissertation Award. Hassanieh developed highly efficient algorithms for computing the Sparse Fourier Transform, and demonstrated their applicability in many domains including networks, graphics, medical imaging and biochemistry. In his dissertation, The Sparse Fourier Transform: Theory and Practice, he presented a new way to decrease the amount of computation needed to process data, thus increasing the efficiency of programs in several areas of computing.
In computer science, the Fourier transform is a fundamental tool for processing streams of data. It identifies frequency patterns in the data, a task that has a broad array of applications. For many years, the Fast Fourier Transform (FFT) was considered the most efficient algorithm in this area. With the growth of Big Data, however, the FFT cannot keep up with the massive increase in datasets. In his doctoral dissertation Hassanieh presents the theoretical foundation of the Sparse Fourier Transform (SFT), an algorithm that is more efficient than FFT for data with a limited number of frequencies. He then shows how this new algorithm can be used to build practical systems to solve key problems in six different applications including wireless networks, mobile systems, computer graphics, medical imaging, biochemistry and digital circuits. Hassanieh’s Sparse Fourier Transform can process data at a rate that is 10 to 100 times faster than was possible before, thus greatly increasing the power of networks and devices.
Hassanieh is an Assistant Professor in the Department of Electrical and Computer Engineering and the Department of Computer Science at the University of Illinois at Urbana-Champaign. He received his MS and PhD in Electrical Engineering and Computer Science at the Massachusetts Institute of Technology (MIT). A native of Lebanon, he earned a BE in Computer and Communications Engineering from the American University of Beirut. Hassanieh’s Sparse Fourier Transform algorithm was chosen by MIT Technology Review as one of the top 10 breakthrough technologies of 2012. He has also been recognized with the Sprowls Award for Best Dissertation in Computer Science, and the SIGCOMM Best Paper Award.
Honorable Mention for the 2016 ACM Doctoral Dissertation Award went to Peter Bailis of Stanford University and Veselin Raychev of ETH Zurich.
In Bailis’s dissertation, Coordination Avoidance in Distributed Databases, he addresses a perennial problem in a network of multiple computers working together to achieve a common goal: Is it possible to build systems that scale efficiently (process ever-increasing amounts of data) while ensuring that application data remains provably correct and consistent? These concerns are especially timely as Internet services such as Google and Facebook have led to a vast increase in the global distribution of data. In addressing this problem, Bailis introduces a new framework, invariant confluence, that mitigates the fundamental tradeoffs between coordination and consistency. His dissertation breaks new conceptual ground in the areas of transaction processing and distributed consistency—two areas thought to be fully understood. Bailis is an Assistant Professor of Computer Science at Stanford University. He received a PhD in Computer Science from the University of California, Berkeley and his AB in Computer Science from Harvard College.
Raychev’s dissertation, Learning from Large Codebases, introduces new methods for creating programming tools based on probabilistic models of code that can solve tasks beyond the reach of current methods. As the size of publicly available codebases has grown dramatically in recent years, so has interest in developing programming tools that solve software tasks by learning from these codebases. Raychev’s dissertation takes a novel approach to addressing this challenge that combines advanced techniques in programming languages with machine learning practices. In the thesis, Raychev lays out four separate methods that detail how machine learning approaches can be applied to program analysis in order to produce useful programming tools. These include: code completion with statistical language models; predicting program properties from big code; learning program from noisy data; and learning statistical code completion systems. Raychev’s work is regarded as having the potential to open up several promising new avenues of research in the years to come. Raychev is currently a co-founder and Chief Technology Officer of DeepCode, a company developing artificial intelligence-based programming tools. He received a PhD in Computer Science from ETH Zurich. A native of Bulgaria, he received MS and BS degrees from Sofia University.
Carnegie Mellon Graduate Earns ACM Doctoral Dissertation Award
Julian Shun has won the 2015 ACM Doctoral Dissertation Award presented by ACM for providing evidence that, with appropriate programming techniques, frameworks and algorithms, shared-memory programs can be simple, fast and scalable. In his dissertation Shared-Memory Parallelism Can Be Simple, Fast, and Scalable, he proposes new techniques for writing scalable parallel programs that run efficiently both in theory and in practice.
While parallelism is essential to achieving high performance in computing, writing efficient and scalable programs can be very difficult. Shun’s three-pronged approach to writing parallel programs that he outlines in his thesis includes:
- proposing tools and techniques for deterministic parallel programming;
- the introduction of Ligra, the first high-level shared-memory framework for parallel graph traversal algorithms; and
- presenting new algorithms for a variety of important problems on graphs and strings that are both efficient in theory and practice.
Shun is a post-doctoral researcher at the University of California, Berkeley, where he was awarded a Miller Research Fellowship. He earned his Ph.D. at Carnegie Mellon University, which nominated him for the ACM Doctoral Dissertation Award. He earned a B.A. in Computer Science from the University of California, Berkeley, where he was ranked first in the 2008 graduating class of computer science students. During the 2013-2014 academic year, he was the recipient of a Facebook Graduate Fellowship.
He will receive the Doctoral Dissertation Award and its $20,000 prize at the annual ACM Awards Banquet on June 11 in San Francisco. Financial sponsorship of the award is provided by Google Inc.
Honorable Mention
Honorable mention for the 2015 ACM Doctoral Dissertation Award went to Aaron Sidford of the Massachusetts Institute of Technology, and Siavash Mirarab of the University of Texas at Austin. They will share a $10,000 prize, with financial sponsorship provided by Google Inc.
In Sidford’s dissertation, Iterative Methods, Combinatorial Optimization, and Linear Programming Beyond the Universal Barrier, he considers the fundamental problems in continuous and combinatorial optimization that occur pervasively in practice, and shows how to improve upon the best-known theoretical running times for solving these problems across a broad range of parameters. Sidford uses and improves techniques from diverse disciplines including spectral graph theory, numerical analysis, data structures, and convex optimization to provide the first theoretical improvements in decades for multiple classic problems ranging from linear programming to linear system solving to maximum flow. Sidford is presently a postdoctoral researcher at Microsoft New England. He received a Ph.D. in Computer Science from the Massachusetts Institute of Technology, which nominated him for this award.
Mirarab’s dissertation, Novel Scalable Approaches for Multiple Sequence Alignment and Phylogenomic Reconstruction, addresses the growing need to analyze large-scale biological sequence data efficiently and accurately. To address this challenge, Mirarab introduces several methods: PASTA, a scalable and accurate algorithm that can align data sets up to one million sequences; statistical binning, a novel technique for reducing noise in estimation of evolutionary trees for individual parts of the genome; and ASTRAL, a new summary method that can run on 1,000 species in one day and has outstanding accuracy. These methods were essential in analyzing very large genomic datasets of birds and plants. Mirarab is currently an Assistant Professor of Electrical and Computer Engineering at the University of California, San Diego. He obtained a Ph.D. in Computer Science from the University of Texas at Austin, which nominated him for this award.
Creator Of Advanced Data Processing Architecture Wins 2014 Doctoral Dissertation Award
Matei Zaharia won the 2014 Doctoral Dissertation Award for his innovative solution to tackling the surge in data processing workloads, and accommodating the speed and sophistication of complex multi-stage applications and more interactive ad-hoc queries. His work proposed a new architecture for cluster computing systems, achieving best-in-class performance in a variety of workloads while providing a simple programming model that lets users easily and efficiently combine them.
To address the limited processing capabilities of single machines in an age of growing data volumes and stalling process speeds, Zaharia developed Resilient Distributed Datasets (RDDs). As described in his dissertation “An Architecture for Fast and General Data Processing on Large Clusters,” RDDs are a distributed memory abstraction that lets programmers perform computations on large clusters in a faulttolerant manner. He implements RDDs in the open source Apache Spark system, which matches or exceeds the performance of specialized systems in many application domains, achieving up to speeds 100 times faster for certain applications. It also offers stronger fault tolerance guarantees and allows these workloads to be combined.
Zaharia, an assistant professor at MIT’s Computer Science and Artificial Intelligence Laboratory (CSAIL), completed his dissertation at the University of California, Berkeley, which nominated him. A graduate of the University of Waterloo, where he won a gold medal at the ACM International Collegiate Programming Contest (ICPC) in 2005, he earned a Bachelor of Mathematics (B. Math) degree. He is a co-founder and Chief Technology Officer of Databricks, the company that is commercializing Apache Spark.
He will receive the Doctoral Dissertation Award and its $20,000 prize at the annual ACM Awards Banquet on June 20 in San Francisco, CA. Financial sponsorship of the award is provided by Google Inc.
Honorable Mention for the 2014 ACM Doctoral Dissertation Award went to John Criswell of the University of Rochester, and John C. Duchi of Stanford University. They will share a $10,000 prize, with financial sponsorship provided by Google Inc.
Criswell’s dissertation, “Secure Virtual Architecture: Security for Commodity Software Systems,” describes a compiler-based infrastructure designed to address the challenges of securing systems that use commodity operating systems like UNIX or Linux. This Secure Virtual Architecture (SVA) can protect both operating system and application code through compiler instrumentation techniques. He completed a Ph.D. degree in Computer Science from the University of Illinois at Urbana-Champaign, which nominated him for this award.
Duchi’s dissertation, “Multiple Optimality Guarantees in Statistical Learning,” explores tradeoffs that occur in modern statistical and machine learning applications. The criteria for these tradeoffs – computation, communication, privacy – must be optimized to maintain statistical performance. He explores examples from optimization, and shows some of the practical benefits that a focus on multiple optimality criteria can bring about. A graduate of the University of California, Berkeley with an M.A. degree in Statistics and a Ph.D. degree in Computer Science, he was also an undergraduate and masters student at Stanford University. He was nominated by UC Berkeley for this award.
Doctoral Dissertation Award Recognizes Young Researchers
Ashish Sharma is the recipient of the ACM Doctoral Dissertation Award for his dissertation “Human-AI Collaboration to Support Mental Health and Well Being.” Sharma developed fundamental advances in natural language processing to positively impact the mental health of many people. Honorable Mentions go to Alexander (Zander) Kelley for his dissertation “Explicit Pseudorandom Distributions for Restricted Models of Computation” toward a PhD earned at the University of Illinois Urbana-Champaign; and Sewon Min for her dissertation “Rethinking Data Use in Large Language Models” toward a PhD earned at the University of Washington.
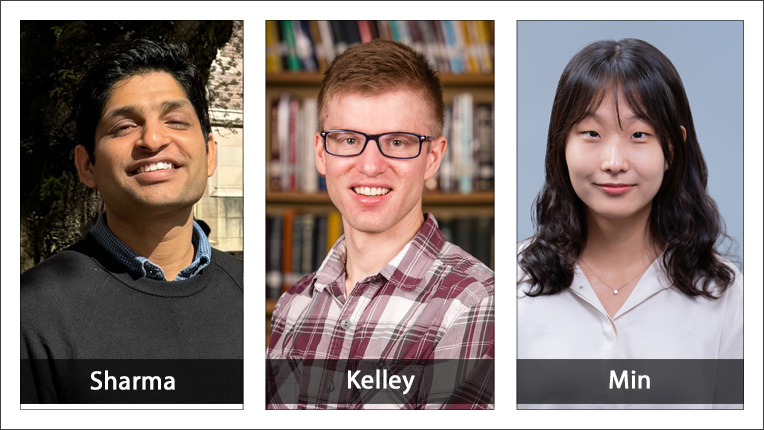
ACM Awards by Category
-
Career-Long Contributions
-
Early-to-Mid-Career Contributions
-
Specific Types of Contributions
ACM Charles P. "Chuck" Thacker Breakthrough in Computing Award
ACM Eugene L. Lawler Award for Humanitarian Contributions within Computer Science and Informatics
ACM Frances E. Allen Award for Outstanding Mentoring
ACM Gordon Bell Prize
ACM Gordon Bell Prize for Climate Modeling
ACM Luiz André Barroso Award
ACM Karl V. Karlstrom Outstanding Educator Award
ACM Paris Kanellakis Theory and Practice Award
ACM Policy Award
ACM Presidential Award
ACM Software System Award
ACM Athena Lecturer Award
ACM AAAI Allen Newell Award
ACM-IEEE CS Eckert-Mauchly Award
ACM-IEEE CS Ken Kennedy Award
Outstanding Contribution to ACM Award
SIAM/ACM Prize in Computational Science and Engineering
ACM Programming Systems and Languages Paper Award -
Student Contributions
-
Regional Awards
ACM India Doctoral Dissertation Award
ACM India Early Career Researcher Award
ACM India Outstanding Contributions in Computing by a Woman Award
ACM India Outstanding Contribution to Computing Education Award
IPSJ/ACM Award for Early Career Contributions to Global Research
CCF-ACM Award for Artificial Intelligence -
SIG Awards
-
How Awards Are Proposed